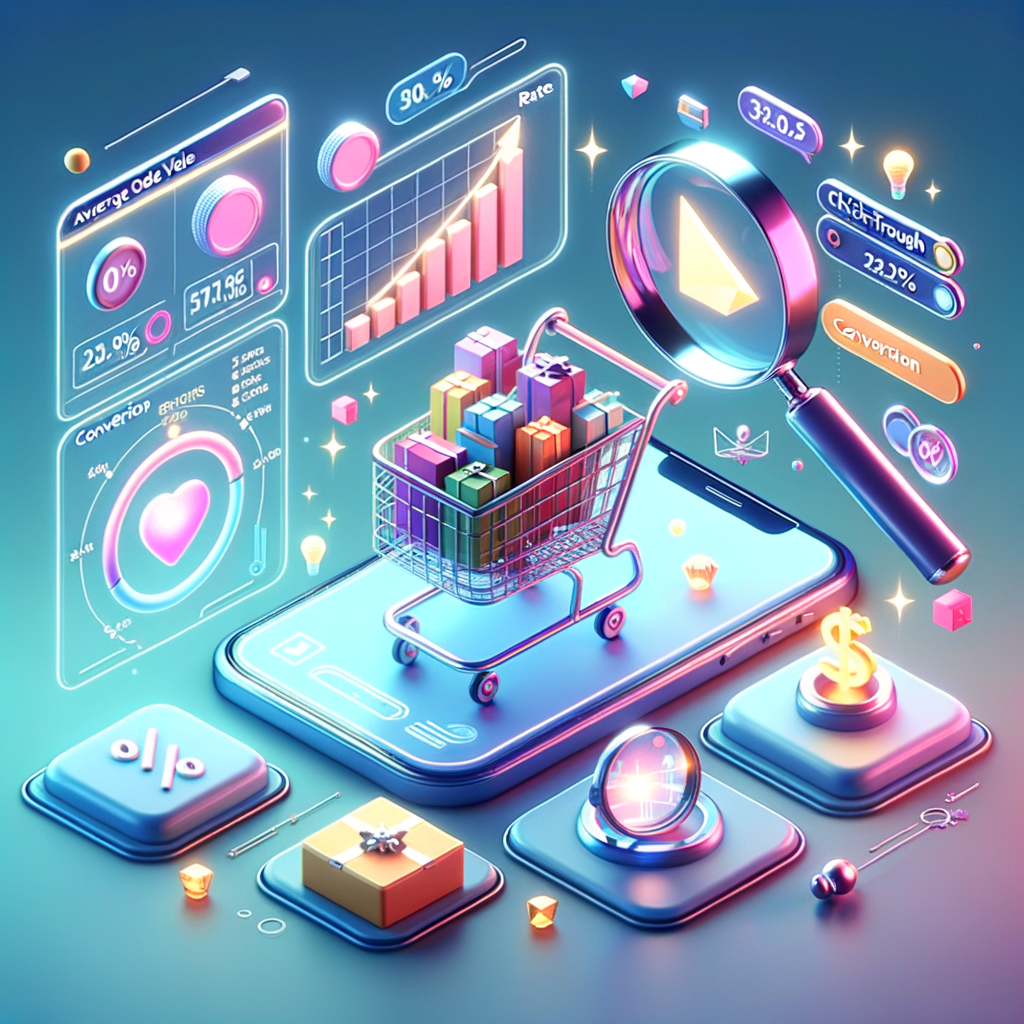
Introduction to AI-Driven Personalized Product Recommendations
In today’s competitive e-commerce landscape, businesses are constantly looking for ways to enhance customer experiences and drive conversions. One powerful solution that has risen to prominence is AI-driven personalized product recommendations.
These intelligent recommendations leverage the power of artificial intelligence and machine learning algorithms to deliver tailored suggestions to each individual customer.
The goal of AI based personalized recommendations is simple – to provide customers with relevant and enticing suggestions based on their unique preferences. This technology analyzes vast amounts of customer data, ranging from purchase history to browsing behavior, to gain a more in-depth understanding of each individual’s likes and interests.
By tapping into this wealth of information, businesses can offer a personalized shopping experience that increases customer satisfaction and drives sales.
So, how does AI-driven personalized product recommendation work in e-commerce? It all starts with sophisticated algorithms that are designed to gather, process, and interpret customer data. These algorithms use techniques such as collaborative filtering, content-based filtering, and reinforcement learning to identify patterns and trends in customer behavior.
By analyzing purchasing patterns, browsing history, and other relevant factors, the system can generate a list of recommended products that a customer is likely to be interested in.
The AI-based recommendation engine considers multiple factors when generating recommendations. This includes factors such as the customer’s browsing habits, past purchases, similar customer behavior, and even real-time trends. By considering these factors, businesses can offer personalized suggestions that align with the customer’s preferences and increase the chances of conversion.
One of the key benefits of AI smart recommendations is the accuracy of the suggestions. With machine learning algorithms constantly fine-tuning and updating based on customer data, the recommendations become more accurate over time. This means that as customers engage with the recommendations and provide feedback, the system can continuously learn and adapt to deliver even more relevant suggestions.
By providing smart recommendations, businesses can significantly enhance the customer experience. Customers are more likely to engage with suggestions that align with their interests, leading to higher conversion rates and average order values.
In addition, providing tailored recommendations can foster customer loyalty, as customers feel that the business understands their needs and provides tailored solutions.
AI based custom recommendations have already shown tremendous success in e-commerce. Major players like Amazon, Netflix, and Spotify have implemented these systems to great effect, driving customer engagement and boosting sales.
However, AI-based recommendations are not limited to large corporations. With the increasing availability and affordability of AI-powered tools, businesses of all sizes can harness the power of customized recommendations to enhance their e-commerce strategies.
As the e-commerce landscape continues to evolve, businesses that leverage AI-based recommendations will stand out from the crowd and deliver personalized shopping experiences that keep customers coming back for more.
Underlying Technology and Algorithms
Personalized AI recommendations rely on sophisticated technologies and algorithms to analyze customer data and generate tailored suggestions. These underlying technologies include machine learning (ML) and deep learning (DL), which enable the system to learn from data and make predictions.
Machine learning, in simple terms, is like teaching a computer to learn from experience, similar to how a child learns from their surroundings. It involves training algorithms on large datasets to identify patterns and make predictions or recommendations based on those patterns.
One frequently employed method for creating tailored suggestions is known as collaborative filtering. This algorithm analyzes user behavior and recommends products based on the preferences of similar users. For example, if two users have similar browsing or purchasing history, the algorithm may suggest items that one user has purchased to the other user.
Content-based filtering is another popular algorithm used in AI recommendations. This algorithm recommends products based on the attributes or characteristics of the items themselves. For instance, if a customer has shown interest in specific product features or categories, the algorithm may suggest similar products that match those preferences.
To train AI models for personalized product suggestions, data from various sources is typically used. These sources can include customer purchase history, browsing behavior, demographic information, and even real-time trends. By combining and analyzing these different data sources, the AI model can gain a more in-depth understanding of each customer’s preferences and make more accurate recommendations.
In addition to collaborative filtering and content-based filtering, other algorithms and techniques, such as reinforcement learning, can also be employed to improve the accuracy and personalization of recommendations. Reinforcement learning involves training the AI model to optimize recommendations based on feedback and rewards from customer interactions.
In essence, the foundational technology and computational methods employed in AI-powered suggestions enable companies to utilize consumer information and present individualized recommendations. By utilizing algorithms like collaborative filtering and content-based filtering, businesses can offer relevant suggestions that align with the individual customer’s interests and increase the likelihood of conversion.
Understanding User Behavior and Preferences
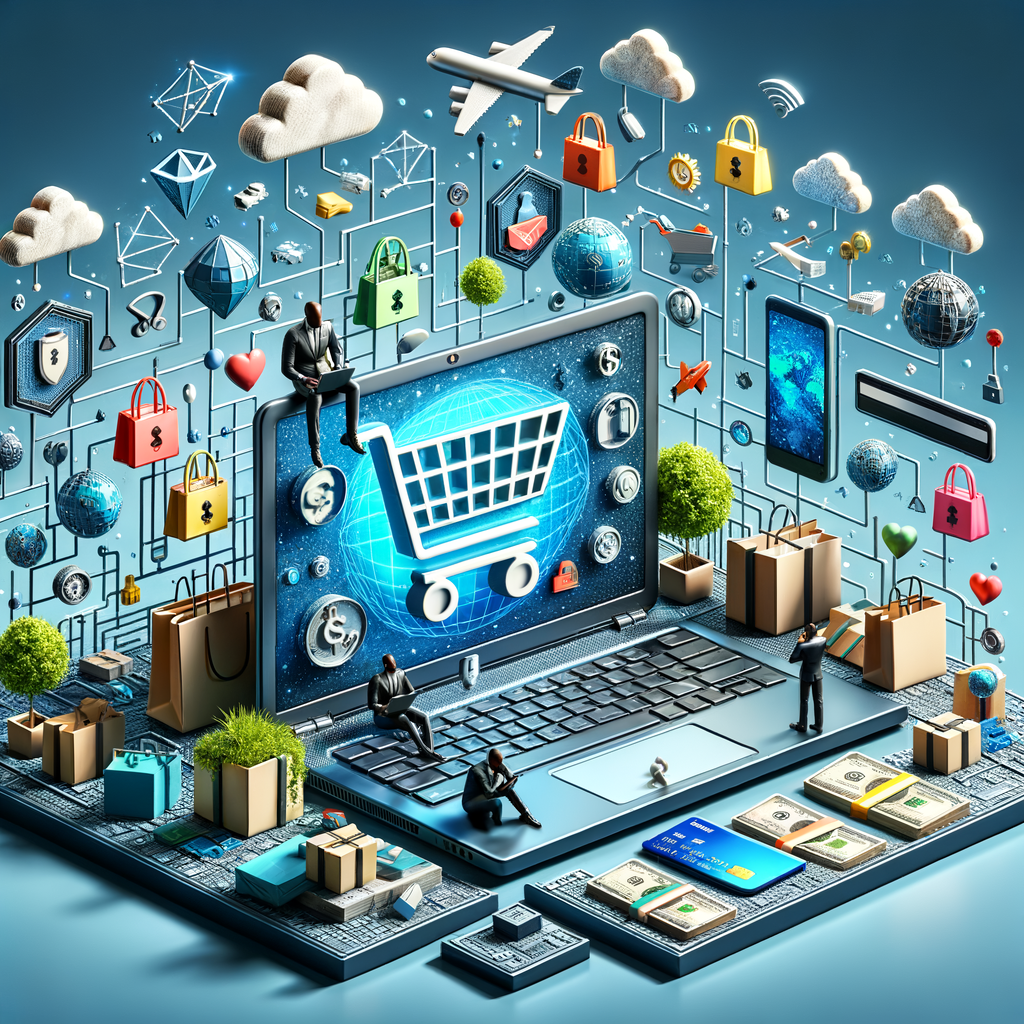
AI’s personalized recommendations rely on understanding user behavior and preferences to provide accurate and tailored suggestions. By analyzing various data sources such as purchase history, browsing behavior, demographics, and even real-time trends, AI can gain insights into individual customer preferences and make recommendations that align with their interests.
One of the key data sources used in providing custom recommendations is the customer’s purchase history. By analyzing the products a customer has previously purchased, AI can identify patterns and preferences. For example, if a customer has previously bought a hiking book, the AI system can infer an interest in outdoor activities and suggest related products such as camping gear or hiking boots.
Browsing behavior is another crucial data source that helps AI systems understand user preferences. By tracking the pages a customer visits, the items they view, and the time spent on each page, AI can gather information about the customer’s specific interests. For example, if a customer spends a significant amount of time looking at cameras on an e-commerce website, the AI system can recommend other camera models or related photography accessories.
Demographic information also plays a role in understanding user behavior and preferences. Factors such as age, gender, location, and income level can provide insights into the types of products a customer may be interested in. For instance, if a customer is a young adult living in a city, the AI system may suggest trendy fashion items or urban lifestyle products that align with their demographic profile.
To identify user preferences, AI algorithms analyze both explicit and implicit signals. Explicit signals include actions such as clicks, searches, and reviews. For example, if a customer clicks on a specific product or leaves a positive review, it indicates a preference for that item. Implicit signals, on the other hand, are more subtle and require the AI system to infer preferences based on observed behavior. For instance, if a customer repeatedly searches for running shoes, it suggests an interest in athletic footwear.
An essential aspect of AI recommendations is their ability to adapt to changing user preferences over time. As customer behaviors and interests evolve, the AI algorithms continuously learn and update the recommendations accordingly. For example, if a customer starts browsing for baby products after becoming a parent, the AI system can adjust and start suggesting baby-related items. By analyzing both historical and real-time data, AI can consistently provide tailored recommendations that stay relevant to the customer’s evolving preferences.
In conclusion, the analysis of explicit and implicit signals allows AI to accurately identify user interests and deliver tailor-made recommendations. Moreover, the ability to adapt to changing preferences over time ensures that the recommendations remain relevant and enhance the customer’s shopping experience.
Why Use AI-Driven Personalization in E-Commerce?
AI-driven personalization in e-commerce brings numerous benefits to businesses and customers alike. By leveraging AI algorithms and recommendation engines, e-commerce platforms can offer personalized experiences that enhance buyer satisfaction, drive conversions, and foster customer loyalty. Here are the key reasons why businesses should embrace AI’s personalization in e-commerce:
- Improved Conversion Rates: AI based recommendations can significantly boost conversion rates by presenting customers with relevant and enticing suggestions. For instance, when John searches for running shoes, the AI system can analyze his browsing behavior and recommend complementary items such as socks and insoles. By offering personalized and relevant product suggestions, businesses can increase the likelihood of customers making a purchase, ultimately leading to higher conversion rates.
- Increased Average Order Value: AI-powered recommendations can encourage customers to add more items to their shopping cart. By utilizing machine learning algorithms to analyze customer data, such as purchase history and browsing behavior, AI can suggest complementary or higher-priced products that align with the customer’s interests. For example, when a customer adds a camera to their cart, the AI system can recommend camera lenses or camera bags, increasing the average order value.
- Enhanced Customer Satisfaction: Customized suggestions enable businesses to deliver a tailored shopping experience that meets the specific needs and preferences of each customer. This level of customization leads to higher client satisfaction, as customers find the products they need more easily, reducing the time spent searching for relevant items.
- Increased Customer Loyalty: When customers receive individualized product suggestions that meet their preferences, they are more likely to feel valued and remain loyal to the business. By continuously analyzing and learning from customer data, AI algorithms can provide recommendations that create a positive and memorable shopping experience. This, in turn, fosters long-term customer loyalty and increases the chances of repeat purchases.
- Improved Customer Experience: By leveraging AI, businesses can create a seamless and intuitive shopping experience for their customers. AI algorithms can analyze and process vast amounts of customer data in real-time, allowing for dynamic and up-to-date product suggestions. From this, customers can easily discover new products that align with their interests and preferences, enhancing their overall shopping experience.
Hence, AI plays a pivotal role in transforming the e-commerce landscape by offering tailored suggestions. By leveraging the power of AI, e-commerce platforms can unlock the full potential of custom recommendations, ultimately leading to business growth and client satisfaction.
Accuracy of AI Recommendations
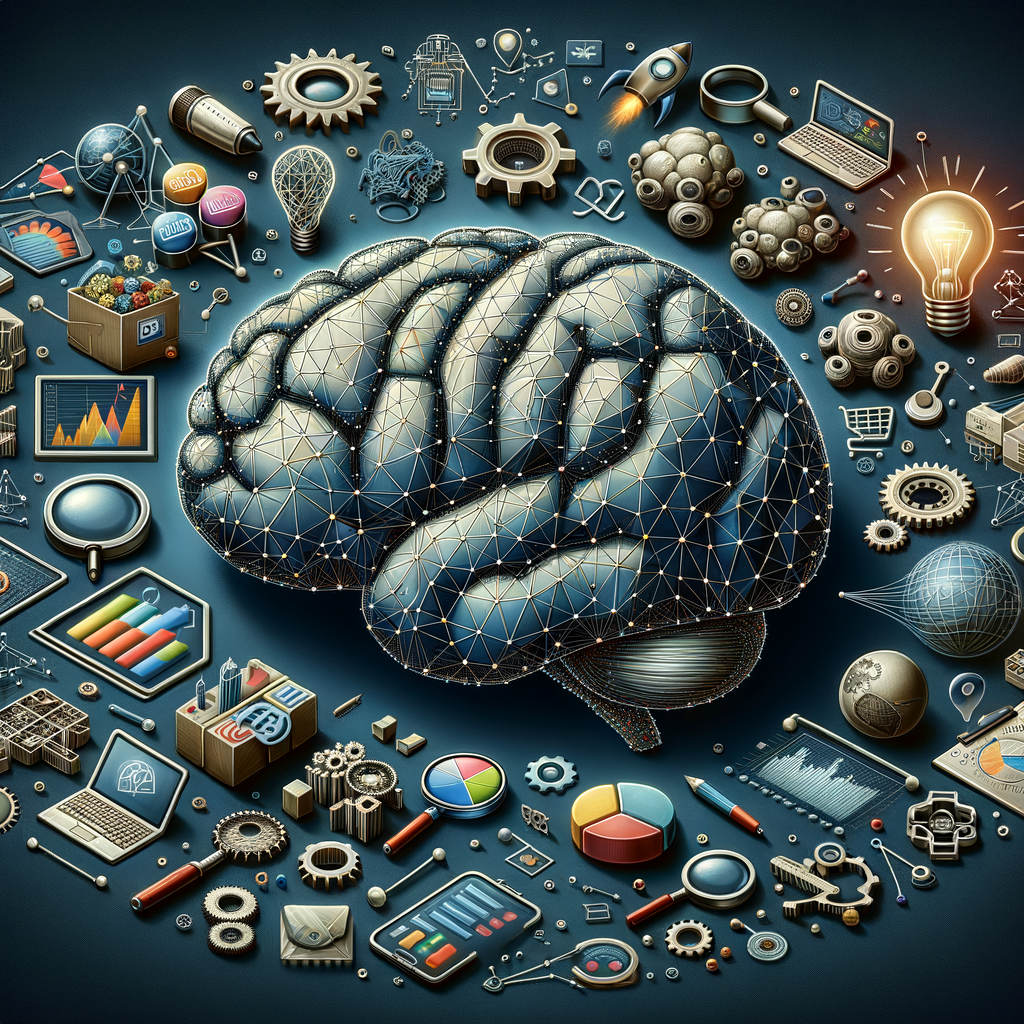
The accuracy of AI’s customized recommendations is a crucial factor in ensuring the success and effectiveness of an e-commerce platform. Several key factors contribute to the accuracy of these recommendations, ultimately determining their relevance and value to customers.
One of the primary contributors to accuracy is the quality and diversity of the customer data that the AI algorithms analyze. The more comprehensive and representative the data, the better the AI system can understand user behavior and preferences. Factors such as purchase history, browsing patterns, demographic information, and explicit customer feedback all play a role in generating accurate recommendations.
However, it is essential to address concerns about data bias and limitations of AI. AI algorithms are designed to learn from historical data, which means they can inadvertently amplify biases present in the data. To mitigate this issue, thorough data analysis and preprocessing techniques should be employed to identify and correct any bias. Additionally, ongoing human oversight is crucial for monitoring and refining the recommendations to ensure fairness and inclusivity.
To further improve the accuracy of AI based recommendations, businesses can implement strategies such as A/B testing. By conducting experiments with different recommendation algorithms, businesses can assess the performance and accuracy of each approach, allowing them to refine their recommendation system continuously. A/B testing provides valuable insights into the effectiveness of different algorithms and helps optimize their performance to deliver more accurate custom recommendations.
Another strategy to enhance accuracy is incorporating user feedback into the recommendation system. By allowing customers to rate and provide feedback on recommended products, the AI algorithms can learn and adjust recommendations based on direct user feedback. This iterative process helps refine the accuracy of the tailored recommendations over time and ensures that they are aligned with the customer’s evolving preferences.
Moreover, machine learning algorithms can be trained to adapt to real-time changes in user behavior and preferences. By analyzing the customer’s interaction with the platform, such as click-through rates, time spent on product pages, and purchase decisions, the AI algorithms can continuously update and fine-tune its unique recommendations.
This dynamic learning process ensures that the recommendations remain accurate and up to date. In conclusion, by addressing concerns about bias, leveraging human expertise, and continuously refining the algorithms, businesses can enhance the accuracy of the AI recommendation systems and unlock their full potential in driving end user satisfaction and increasing conversion rates.
How Artificial Intelligence Differs from Traditional Methods
When it comes to personalized recommendations in e-commerce, Artificial Intelligence (AI) offers a significant advantage over traditional methods. AI based recommendations are powered by advanced algorithms and machine learning techniques that enable them to provide accurate and tailored suggestions to customers based on their preferences and behavior. Here’s how AI differs from other traditional recommendation methods:
- Flexibility and Adaptability: Unlike traditional methods that rely on pre-defined rules and static algorithms, AI-powered recommendation engines can adapt and learn over time. They can analyze vast amounts of customer data, including purchase history, browsing behavior, and demographic information, to generate accurate and customized recommendations. AI algorithms can continuously update and fine-tune themselves based on real-time user interactions, ensuring that the recommendations remain relevant and up to date.
- Complexity and Contextual Understanding: AI algorithms possess a higher level of complexity and contextual understanding compared to traditional methods. They can analyze multiple data points simultaneously and identify complex patterns and correlations in customer behavior. For example, they can consider factors such as seasonality, current trends, and social media influence to provide unique recommendations that align with the customer’s preferences and current needs.
- Scalability and Efficiency: AI-based recommendation systems are highly scalable and efficient. They can handle large datasets, process real-time data, and generate recommendations in a fraction of the time that traditional methods would require. This scalability ensures that AI recommendations can handle the growing volume of customer data and provide personalized suggestions to a large customer base without compromising accuracy or speed.
- Improved Customer Experience: AI-powered recommendations significantly enhance the overall customer experience in e-commerce. By delivering personalized suggestions that align with the customer’s preferences and needs, AI recommendation systems create a personalized shopping experience. This personalized approach leads to higher client satisfaction, increased engagement, and increased customer loyalty. The ability to provide tailor-made recommendations based on real-time data improves the accuracy and relevance of suggestions, ultimately leading to a more personalized and satisfying shopping journey for customers.
- Continuous Learning and Optimization: AI algorithms are designed to continuously learn and optimize themselves based on feedback and customer interactions. Unlike traditional methods, which rely on static rules and algorithms, AI recommendation systems can adapt and improve over time. By analyzing user feedback, purchase decisions, and behavior patterns, AI algorithms can identify trends, preferences, and customer segments. This continuous learning process allows businesses to refine their recommendation strategies and deliver more accurate and personalized suggestions, ultimately leading to improved conversion rates and average order value.
In summary, AI recommendations offer several advantages over traditional methods in e-commerce. The flexibility and adaptability of AI algorithms, their ability to understand contextual information, scalability and efficiency, improved customer experience, and continuous learning and optimization capabilities make AI recommendations a powerful tool for businesses.
Successful Implementations and Best Practices
The implementation of AI’s personalized recommendations has proven to be highly successful in various industries, particularly in e-commerce. Many companies have leveraged AI algorithms and recommendation engines to enhance the customer experience, improve conversion rates, and drive customer loyalty. Let’s take a look at some notable examples of successful implementations and explore best practices for integrating recommendations into e-commerce platforms.
Notable Examples of Successful Implementations:
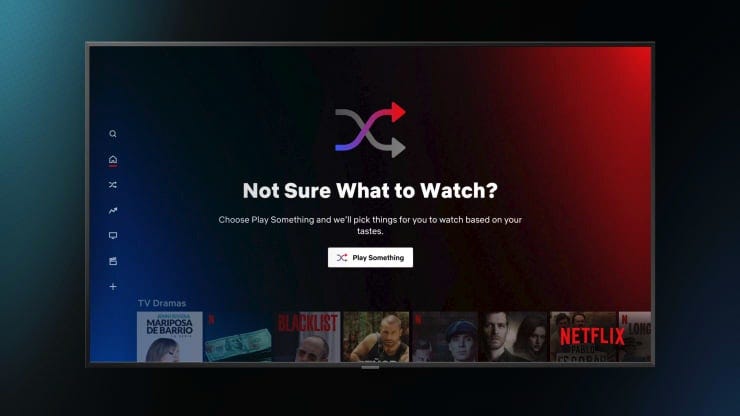
- Amazon: As one of the pioneers in AI recommendations, Amazon has implemented a highly effective custom recommendation system. By analyzing customer data such as browsing history, purchase history, and demographic information, Amazon’s recommendation engine suggests products that align with each individual’s preferences. This has significantly increased customer engagement, conversion rates, and average order value.
- Netflix: Known for its accurate movie and TV show recommendations, Netflix relies heavily on AI algorithms to provide personalized suggestions to its users. By analyzing user behavior, viewing history, and ratings, Netflix offers a tailored streaming experience, keeping users engaged and satisfied. The success of Netflix’s recommendation system is evident in its high customer retention rates and growing subscriber base.
Best Practices for Implementing AI-Based Personalized Product Recommendations:
- Set Clear Goals: Before implementing AI recommendations, it is crucial to define clear objectives. Determine what specific business goals you aim to achieve with custom recommendations, such as increasing conversion rates, average order value, or customer retention. Setting clear goals helps to align implementation strategies and measure success effectively.
- Ensure Data Quality and Accuracy: AI algorithms heavily rely on accurate and high-quality data. Ensure that your customer data is clean, up-to-date, and reliable. Regularly update and maintain your data to ensure the accuracy of recommendations. Additionally, consider leveraging external data sources and integrating customer feedback to further enhance the quality of recommendations.
- Consider Ethical Considerations: When implementing AI recommendations, it is essential to prioritize ethical considerations. Transparently inform users about the use of their data and provide them with options to control their privacy settings. Avoid using sensitive or personal information without explicit consent. Upholding ethical standards maintains user trust and helps build long-term customer relationships.
- Continuously Optimize and Test: Implementing AI recommendations is an ongoing process. Continuously analyze and optimize your recommendation algorithms based on user feedback and performance metrics. Conduct A/B testing to evaluate the effectiveness of different recommendation strategies. This iterative approach ensures that your recommendations remain accurate and improve over time.
- Personalize the Shopping Experience: To provide the best customer experience, tailor your recommendations to individual preferences and needs. Consider factors such as browsing behavior, purchase history, and demographics. Incorporate contextual information such as seasonality, trends, and social media influence. By delivering highly optimized and tailored recommendations, you can create a unique shopping experience that resonates with your customers.
In conclusion, integrating AI-driven recommendations into e-commerce platforms has proven to be highly effective. By following best practices, including setting clear goals, ensuring data quality and accuracy, considering ethical considerations, continuously optimizing and testing, and personalizing the shopping experience, businesses can unlock the full potential of AI recommendations and achieve significant improvements in conversion rates, client satisfaction, and loyalty.
Integration and Measurement of Success
To fully harness the power of AI’s personalized recommendations, e-commerce businesses need to seamlessly integrate recommendation systems into their existing platforms and infrastructure. Here’s how businesses can effectively integrate AI and measure the success of their recommendation systems.
Integration:
- Platform Integration: E-commerce businesses can integrate AI recommendation engines into their platforms by leveraging APIs or integrating with third-party AI service providers. This allows businesses to tap into the power of AI without the need for extensive internal development.
- Data Integration: Integration of customer data is crucial for accurate and tailor-made recommendations. Businesses should ensure that their recommendation systems are seamlessly connected to their customer databases, including purchase history, browsing behavior, and demographic information. This integration allows AI algorithms to analyze customer data and deliver customized recommendations.
- User Interface Integration: AI-based recommendations should be seamlessly integrated into the user interface of an e-commerce platform to provide a personalized shopping experience. This can be achieved through recommendation widgets, personalized product carousels, or individualized product pages based on user preferences.
Measurement of Success:
- Click-through Rate (CTR): One of the key metrics to measure the success of AI recommendations is the CTR. It indicates the percentage of users who click on recommended products. A higher CTR suggests that the recommendations are engaging and capturing users’ attention.
- Conversion Rate: The conversion rate measures the percentage of users who make a purchase after interacting with the recommended products. A higher conversion rate indicates that the recommendations are effectively influencing users’ buying decisions and driving sales.
- Revenue: One of the ultimate measures of success is the impact AI recommendations have on revenue generation. By tracking the revenue attributed to the recommended products, businesses can determine the effectiveness of their recommendation systems in driving sales and increasing revenue.
- Average Order Value (AOV): A higher AOV suggests that the recommended products are influencing users to make a purchase and encouraging them to spend more. By tracking the AOV of users who interact with the recommended products, businesses can evaluate the impact of customized recommendations on increasing the value of each transaction.
- Customer Satisfaction: Gathering customer feedback through surveys or reviews can provide insights into the user satisfaction levels achieved through providing unique recommendations. High consumer satisfaction indicates that the recommendations are relevant, useful, and enhancing the overall shopping experience.
- Repeat Purchases and Customer Loyalty: Tracking the number of repeat purchases and customer loyalty can help businesses measure the success of AI recommendations in terms of customer retention. If users are consistently returning to make additional purchases based on the recommendations, it indicates that the system is effectively building customer loyalty.
By measuring these key metrics, e-commerce businesses can gain a comprehensive understanding of the success of their AI based personalized product recommendation systems. This data enables businesses to make data-driven decisions, optimize their recommendation algorithms, and continue delivering personalized experiences that drive customer engagement, loyalty, and revenue growth.
AI Product Recommendation Tools
AI product recommendation tools play a pivotal role in helping e-commerce businesses leverage the power of AI based custom recommendations. These tools employ advanced algorithms and machine learning techniques to analyze and understand customer data, enabling businesses to offer personalized and relevant product suggestions. Let’s take a look at some popular AI-powered tools available in the market today.
- Salesforce Einstein: Salesforce Einstein is an AI-powered platform that offers a range of features, including personalized product recommendations. Leveraging machine learning algorithms, Einstein analyzes customer data, such as browsing behavior and purchase history, to understand individual preferences and deliver personalized experiences. It also provides businesses with insights to optimize their recommendation strategies and improve customer engagement.
- Adobe Sensei: Adobe Sensei is an AI and machine learning platform that offers various solutions for e-commerce businesses, including AI-powered product recommendation capabilities. By utilizing data from various sources, such as customer interactions, Sensei provides customized recommendations to enhance the shopping experience. With its deep learning algorithms, Sensei can also predict customer behavior and suggest relevant products, increasing conversion rates and end user satisfaction.
- Google Cloud Recommendations AI: Google Cloud Recommendations AI is a fully managed AI service that helps businesses deliver highly customized recommendations. By utilizing historical customer data, preferences, and real-time user behavior, Recommendations AI generates accurate and relevant recommendations. Its integration with Google Cloud’s infrastructure ensures scalability and flexibility for e-commerce businesses.
- RichRelevance: RichRelevance is an AI-powered personalization platform that enables businesses to create personalized experiences for their customers. Its recommendation engine analyzes customer data in real-time, including purchase history and browsing behavior, to provide accurate and dynamic product suggestions. RichRelevance also offers additional features, such as A/B testing and targeting algorithms, to optimize recommendation strategies and drive conversions.
- Dynamic Yield: Dynamic Yield is an AI-based personalization platform that offers a suite of features, including advanced product recommendations. By analyzing customer data in real-time, Dynamic Yield can deliver tailor-made recommendations based on user behavior and preferences. Its AI-powered algorithms can adapt to changing customer preferences and optimize recommendations for different segments of users, resulting in higher conversions and buyer satisfaction.
These are just a few examples of AI product recommendation tools available in the market. Each tool offers unique features and capabilities, allowing businesses to tailor their recommendation strategies to their specific needs. When choosing an AI recommendation tool, businesses should consider factors such as integration capabilities, scalability, accuracy of recommendations, data privacy, and customer support.
Conclusion
In conclusion, AI based personalized product recommendations have revolutionized the way e-commerce businesses engage with their customers. By leveraging advanced algorithms and machine learning techniques, businesses can now provide personalized shopping experiences that increase customer engagement, conversion rates, and average order value.
While we are on the e-commerce topic, are you interested in getting to know some cool AI tools available to make your online business drive sales? Read all about the Top 20+ AI Tools Available to achieve your online business goals.
The underlying technology and algorithms behind AI’s recommendations enable businesses to understand user behavior and preferences at a granular level. By analyzing customer data such as browsing history, purchase patterns, and demographics, businesses can deliver accurate and relevant product suggestions that resonate with individual customers. This level of personalization not only enhances the customer experience but also builds customer loyalty and satisfaction.
The accuracy of AI recommendations sets them apart from traditional methods. The use of artificial intelligence allows businesses to constantly learn and adapt to changing customer preferences and behaviors. With each customer interaction, the recommendation engine becomes more refined and effective in predicting and suggesting products. This ultimately leads to higher conversion rates and increased revenue.
Successful implementations of AI-based recommendation systems have shown significant improvements in business performance. Companies that have embraced customizing recommendations have experienced higher sales, improved customer satisfaction, and increased customer retention. These success stories highlight the power of AI personalization and its ability to drive business growth and success in the competitive e-commerce landscape.
When implementing AI personalization, businesses should focus on integration and measurement of success. Integrating the recommendation system seamlessly into the e-commerce platform ensures a smooth customer experience and maximizes the impact of customized recommendations. Additionally, businesses should continuously monitor and measure the performance of the recommendation engine to identify areas for improvement and optimize the system accordingly.
As technology continues to advance, the future potential of AI based personalization in e-commerce is limitless. The increasing availability of data, advancements in machine learning algorithms, and the rise of artificial intelligence will further enhance the capabilities of recommendation systems. This will enable businesses to provide even more personalized and targeted product suggestions, leading to higher conversion rates, increased average order value, and improved customer loyalty.
Sources
- https://www.bloomreach.com/en/blog/2022/why-product-recommendations-are-key-to-winning-with-e-commerce-personalization
- https://www.coveo.com/blog/personalized-product-recommendations-in-ecommerce/
- https://www.convertcart.com/blog/ecommerce-personalization-examples
- https://www.optimonk.com/ecommerce-personalization/
- https://www.algolia.com/blog/ux/what-are-personalized-recommendations-and-how-can-they-boost-engagement-and-conversion/
Recent Posts
In this blog post, I delve into how AI is used in customer analytics and user behavior analysis, uncovering the potential of customer segmentation, personalization, product recommendations, and...
AI-powered humanoid robots that can do house chores for us may soon become a reality. And while we're waiting for that tech, we can mess around with cheaper AI robots that can do several tasks. But...